Nofima: vaccine reactions and innate resistance in Salmon
Aquaculture is the name given to the farming of salt water and freshwater fish and marine animals. It is a rapidly growing division of farming across the world; reports from the UN’s FAO suggest that it has been growing more rapidly than any other area of animal food production.
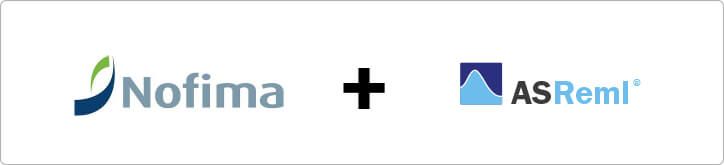
A report published in September 2009 suggested that farm-reared fish accounted for 50% of world fish consumption.
This rise in aquaculture production isn’t much of a surprise when you consider the emphasis on the health-giving properties of fish (particularly Omega-3 from oily fish) and the subsequent encouragement for people to eat more oily fish; and it’s certainly good news for the aquaculture producers across the world. However, growth of this level in any industry, whilst exciting and positive in many ways, also brings with it its own complications. The complexities of all types of farming mean that research and understanding is vital in helping the industry to grow safely as well as rapidly. This is particularly important if the current consumption levels of fish continue: the FAO predict that we will need an extra 27 million tonnes of fish by 2030 to meet the demand.
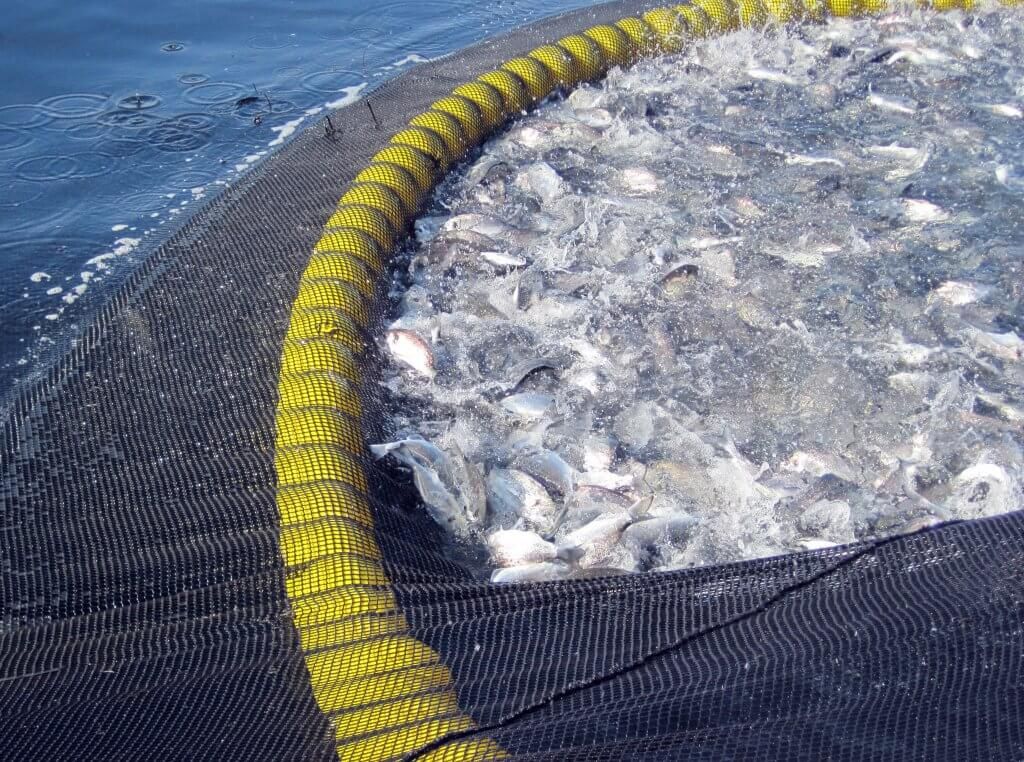
Any farmer will tell you of the importance of vaccination in controlling disease, and this is no different for fish farmers, or specifically Atlantic salmon farmers. Vaccinations against diseases such as furunculosis, vibriosis, cold-water vibriosis, winter ulcer and infectious pancreatic necrosis, which could otherwise decimate a farm, are vital in protecting the fish and farms and controlling these diseases in farmed Atlantic salmon.
However, vaccinations can cause reactions at the injection site such as adhesions and discoloration, reduced appetite and growth, and in severe cases a downgrading of the carcass at market. Not only can this have an economic impact on the salmon farms, but also may create a negative perception of the farms with the general public. As with all aspects of farming the public is concerned not just with the food in question, but also of the welfare of the production animals, which means that breeding companies need to be sure of the actual physical health and safety of their fish.
As with many aspects of farming and agriculture, all aspects of aquaculture are the subject of scientific research to enable better understanding and improved planning for the future, including prevention of disease and improved fish health. The results from challenge tests have indicated substantial genetic variation in disease resistance to a variety of bacterial and viral diseases (including some of those listed earlier), significant but relatively low genetic correlations among the different diseases and a high genetic correlation between the survival of non-vaccinated pre-smolts (smolts are young salmon as they migrate from freshwater to the sea) and the survival of older non-vaccinated full and half sibs under natural break-outs of the diseases. With this in mind Atlantic salmon breeding companies are looking at both the development of efficient vaccinations and the breeding of fish with higher disease resistance.
The results from the challenge test research may suggest that genetic variation in immune systems might lead to genetic variation in innate disease resistance and variation in reactions to the vaccinations. One question researchers in this area need an answer to is whether there is significant genetic correlation between vaccine reactions and innate resistance, as this could mean a change of vaccine dose or a breeding programme to produce fish with the lowest reaction rate to vaccines and the highest natural resistance to diseases.
And it is these issues that a recent project, undertaken by researchers from [Nofima Marin](http://www.nofima.no/marin/en), the [Norwegian School of Veterinary Scienc](http://www.veths.no/)e and [Aqua Gen AS](http://www.aquagen.no/En/Specialfolder/Latest_News/) and funded by the [Research Council of Norway](http://www.forskningsradet.no/servlet/Satellite?c=Page&cid=1177315753906&p=1177315753906&pagename=ForskningsradetEngelsk%2FHovedsidemal) (published in the Elsevier journal Aquaculture 287 (2009) 52-58) has looked at.
Atlantic salmon were taken from farmed strains and from offspring of wild salmon, and reared at different farms. Data was collected on sex, size (at different stages), adhesion scores and melanin scores of fish at harvest size and survival data from challenge tests of their full- and halfsibs. The data collected was analysed using [ASReml](https://www.vsni.co.uk/software/asreml) to fit a multivariate linear mixed animal model to obtain estimates for the variance and covariance components for the random effects of the studied traits. A single trait sire and dam model, which included the same fixed effects was used to obtain across year-class heritability estimates (using an alpha version of [ASReml 3](https://www.vsni.co.uk/software/asreml)).
Results from the project indicate that the severity of vaccine injuries in Atlantic salmon could be reduced through selective breeding. Additionally the relatively high correlation that was found between adhesion scores and melanin scores show that there is unlikely to be a need to select against both of these traits. The researchers warned that including an additional breeding trait objective would reduce the genetic gain for other traits, and therefore recommended that other solutions to the vaccine injuries be found, such as improved vaccines or vaccination procedures. The results also indicated a negative genetic correlation between body weight and adhesion or melanin score; however, these estimates are most likely biased towards the negative end of the scale, since vaccine injuries are expected to reduce growth rate for non-genetic reasons. The study also indicated “no genetic association between resistance to furunuculosis and ISA (infectious salmon anaemia) and vaccine injuries”, which suggest that the vaccine injuries are caused by other aspects of the immune system, not associated with the genetic variation in innate resistance to these diseases.
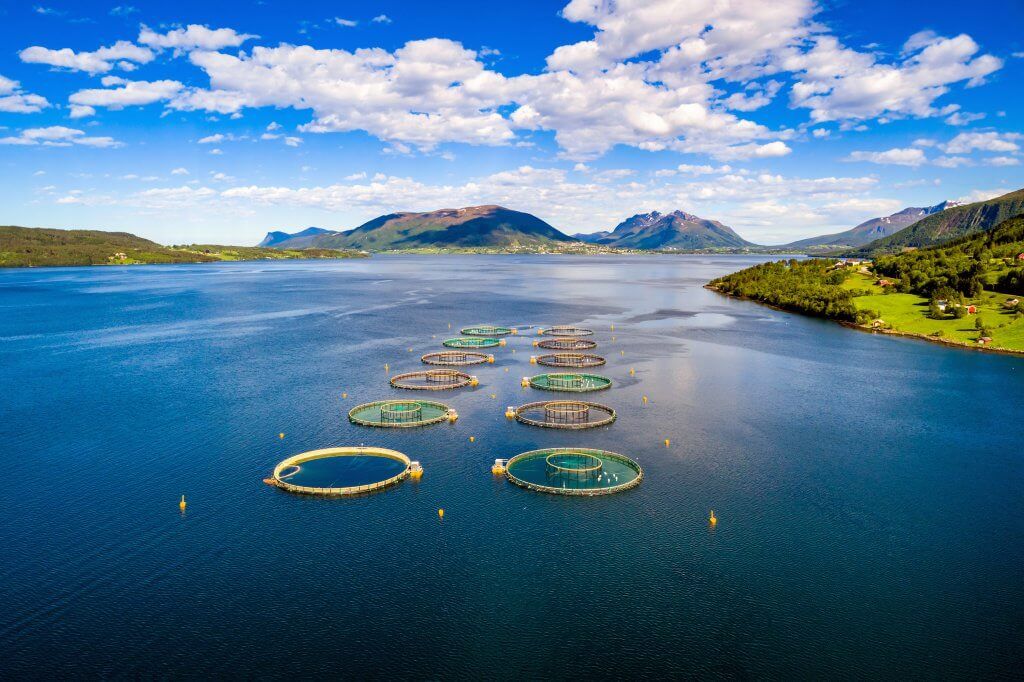
Research of this kind is extremely important to the aquaculture industry to enable it to continue to develop, expand and improve, not just the industry, but the procedures, processes and systems associated with it. ASReml is widely respected and used in situations such as this.
Our thanks to [Dr Bjarne Gjerde](http://www.nofima.no/marin/en/person/bjarne.gjerde) of [Nofima Marin](http://www.nofima.no/marin/en) for his help with this story.